AI in Risk: Transforming Risk Management for the Future
In the evolving landscape of Financial Risk Management, Artificial Intelligence (AI) can be a game changer. Organizations exposed to various kinds of risks are rapidly embracing AI to improve decision-making, streamline risk assessments, and adapt to volatile markets. The transformation AI brings is not just a technological leap; it reshapes how Risk Managers provide value to their firm, from predictive risk analytics to dynamic stress testing.
In this bulletin, we will explore the fundamentals of AI in Risk Management, how it’s being integrated into Financial Risk Management, real-world applications from top Firms, the potential drawbacks and a glimpse into the future of AI that could redefine Risk Management.
Basics of AI
Artificial Intelligence (AI) refers to the use of machines and algorithms designed to replicate human cognitive functions, such as learning, reasoning, and problem-solving. It encompasses a range of advanced techniques, including machine learning (ML), natural language processing (NLP), and robotic process automation (RPA), which together enable systems to process vast amounts of data, recognize patterns, and make informed predictions.
In the realm of Risk Management, AI enhances and refines traditional models in several key ways:
1. Machine Learning (ML): Machine learning algorithms are used to analyze extensive historical data, identifying patterns and relationships that may be too subtle or complex for traditional statistical models. This helps in predicting future risk scenarios with greater accuracy, allowing firms to anticipate potential market fluctuations and stress events.
2. Natural Language Processing (NLP): NLP enables systems to extract valuable insights from unstructured data sources, such as financial news, social media, and earnings reports. By analyzing sentiment and trends in real-time, NLP provides deeper, more nuanced understanding of market movements and investor sentiment, which can inform risk strategies.
3. Robotic Process Automation (RPA): RPA streamlines routine, manual processes, such as risk reporting and data aggregation. By automating these tasks, RPA not only increases efficiency but also improves accuracy, freeing up human analysts to focus on higher-level strategic decisions.
Together, these AI-driven capabilities can make Risk Management faster, more accurate, and more responsive to real-time market conditions, providing Firms and its Risk Managers with a competitive edge in navigating complex and volatile markets.
AI Integration into Risk Management
Integrating AI into Risk Management is transforming the way Risk Managers approach critical tasks, from Value-at-Risk (VaR) calculations and credit risk assessments to liquidity management and operational oversight. AI technologies have the potential to empower Risk Managers with deeper insights and more precise, proactive risk assessments.
A. Data-driven Decision Making: AI models will allow Risk Managers to base decisions on comprehensive, real-time information across risk domains, drawn from vast and diverse data sources.
- Enhanced Data Processing: AI algorithms will enable the ability to seamlessly integrate structured and unstructured data—such as market indicators, credit profiles, cash flow records, and operational metrics—providing a comprehensive view of risk factors that influence overall exposure.
- Real-time Insights: Continuous data analysis using AI will enable Risk Managers to receive up-to-the-minute insights, will allow them to respond swiftly to changing risk conditions, whether in market shifts, borrower credit status, liquidity needs, or operational vulnerabilities.
- Customized Risk Profiles: AI enabled advanced analytics will facilitate tailored risk assessments based on each institution’s unique exposure and risk appetite, ensuring a more personalized and relevant approach to Risk Management.
B. Automation of Routine Analysis: AI automates repetitive risk-related tasks—such as report generation, preliminary analysis, and threshold monitoring—freeing Risk Managers to focus on developing tailored solutions and strategic responses.
- Efficiency Gains: Automation reduces the time required for routine tasks, AI enablement of automation will allow firms to respond more quickly to client needs and shifting market dynamics.
- Error Reduction: Automated processes minimize human error, ensuring consistent and reliable outputs, which is crucial for maintaining accuracy in high-stakes risk and compliance environments.
- Resource Optimization: With AI handling routine analyses, human resources will be redirected toward strategic initiatives, stimulating breakthrough ideas and fostering more value-driven services.
C. Predictive Analytics: AI-powered predictive analytics will offer the ability to anticipate potential shifts across various risk categories, enabling proactive measures and advanced risk mitigation.
- Market Risk Forecasting: AI-driven analysis of historical and real-time data will be able to detect trends that signal potential market volatility, allowing Risk Managers to prepare and adjust strategies proactively.
- Credit Risk Projections: AI models have the potential to identify early warning signs in borrower behavior or economic indicators, enabling timely interventions to mitigate credit risk.
- Liquidity Demand Forecasting: AI models will offer predictive and analytic abilities to assess cash flow and funding requirements, helping firms manage liquidity risk proactively to ensure consistent capital availability.
- Operational Risk Monitoring: AI has the potential to detect patterns indicative of operational disruptions, such as system failures or fraud risks, allowing Risk Managers to implement preventive actions to maintain continuity and compliance.
Growing Momentum in AI-Driven Market Risk Innovations
As demonstrated by the steady rise in intellectual property (IP) filings citing a combination AI and Risk domains between 2015 and 2024, the integration of AI into Market Risk, Credit Risk, Liquidity Risk and Operational Risk processes is accelerating at an unprecedented pace. This trend underscores the growing recognition of AI’s transformative potential in the financial sector, particularly in areas like risk modeling, predictive analytics, and scenario planning.
The chart shows that the number of IP filings more than doubled from 2015 to 2024, reflecting how firms are not only adopting AI but also securing new technologies and processes to gain a competitive edge. The significant jump in filings from 2020 onward aligns with increased demand for real-time risk insights and more sophisticated stress testing following major global market disruptions, including the COVID-19 pandemic and heightened geopolitical tensions.
This innovation surge sets the stage for real-world applications of AI in Risk Management, where advanced algorithms are reshaping how financial advisors manage and mitigate risks. Below, we explore four practical examples of how AI is being leveraged in this space.
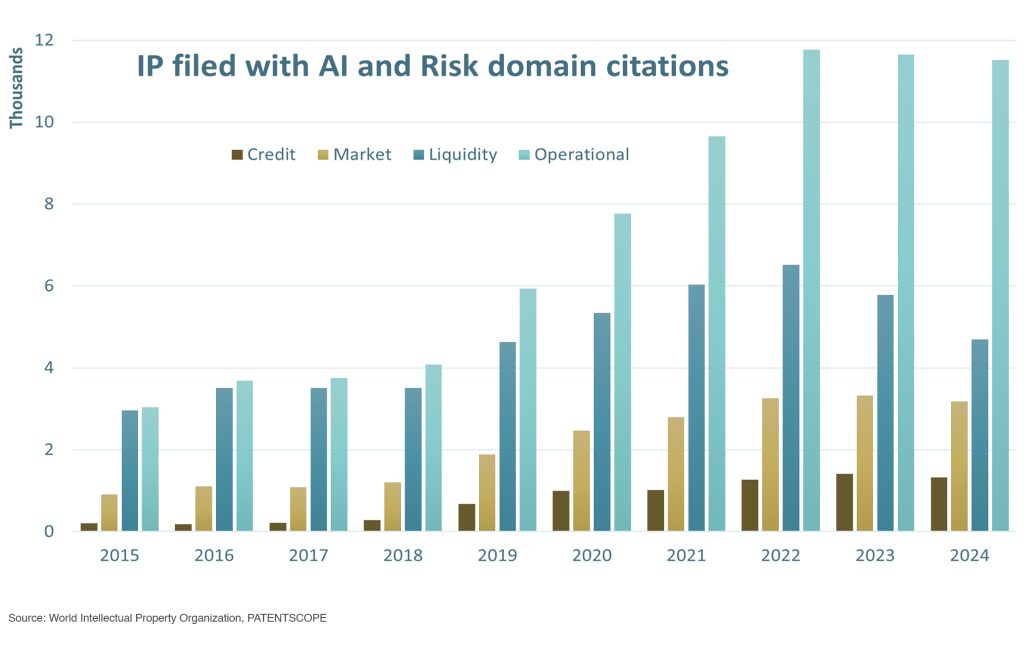
Real-World Applications of AI in Risk
AI has the potential to become a transformative force in Risk Management, enabling Firms and its Risk Managers and risk-exposed firms to optimize risk assessment and strengthen decision-making across all risk domains. Below are key examples of how AI is on this path and how it is being applied in Risk Management, along with insights into the technologies that are reshaping traditional approaches.
A. AI-Driven Scenario Analysis and Stress Testing:
BlackRock’s Aladdin Platform: Leveraging AI, particularly machine learning (ML) and natural language processing (NLP), Aladdin models extreme market scenarios, such as rapid interest rate changes or geopolitical shocks. Aladdin’s models continuously adapt based on real-time data, making them highly responsive to new market conditions.
- ML: Uncovers non-linear asset relationships and refines models based on new data.
- NLP: Analyzes market news and economic reports to adjust stress test parameters.
- Dynamic Risk Simulation: Provides constantly updated simulations reflecting the latest market shifts.
This AI-driven approach helps hedge fund managers better prepare for market volatility, protecting portfolios from potential losses.
B. Liquidity Risk Management Using AI-Enhanced Predictive Analytics:
JPMorgan Chase’s Liquidity Management Platform: Utilizing machine learning and predictive analytics, JPMorgan’s system assesses patterns in transaction history and market conditions to forecast liquidity needs. This allows the bank to maintain optimal liquidity buffers and avoid shortfalls during periods of increased demand. The technologies implemented in the systems are as follows;
- Predictive Analytics: Analyzes transaction volumes and cash flow trends to project short-term and long-term liquidity requirements.
- ML Algorithms: Continuously refine models based on evolving patterns in client transactions and market shifts.
- Anomaly Detection: Identifies irregularities that may signal future liquidity stress, allowing proactive responses.
This AI-based solution enables Risk Managers to better manage liquidity risk and ensure reliable capital access during fluctuating market conditions.
C. AI-Driven Credit Risk Analysis and Early Warning Systems:
Wells Fargo’s Credit Risk Platform: By leveraging machine learning, NLP, and real-time analytics, the platform continuously evaluates credit risk by analyzing borrower behavior, financial data, and market trends. These insights help Wells Fargo proactively adjust credit policies and reduce exposure to high-risk borrowers.
- ML for Credit Scoring: Refines credit scoring models based on historical and current borrower data.
- NLP: Analyzes financial reports and industry news to identify sector-specific risks affecting borrowers.
- Early Warning Systems: Detects early signs of default risk, enabling the bank to adjust lending terms and credit exposure.
Wells Fargo’s platform provides actionable insights to mitigate credit risk effectively, safeguarding financial stability.
D. Operational Risk Detection and Fraud Prevention:
HSBC’s Operational Risk Platform: Employing machine learning, anomaly detection, and NLP, HSBC’s platform monitors transactional data and system activity to detect potential fraud and operational failures.
- Anomaly Detection: Identifies unusual transaction patterns that may indicate fraud or cybersecurity threats.
- ML Algorithms: Adapt to new types of operational risks, such as evolving fraud tactics.
- NLP for Risk Intelligence: Scans regulatory updates and internal reports to ensure the firm is aware of potential vulnerabilities.
This AI-based approach strengthens HSBC’s operational resilience by providing early detection of risks and reducing the potential for costly disruptions.
Cons of AI in Risk Management
While the integration of artificial intelligence (AI) in Risk Management brings many advantages, it’s vital to consider the potential drawbacks. Here are some nuanced challenges that go beyond the common concerns, offering deeper insights into the issues at hand:
1. Model Risk: Many AI models operate under the assumption that historical patterns will continue into the future. This can be problematic in dynamic markets where economic, political, and social factors abruptly shift relationships between variables. As firms increasingly depend on complex algorithms, how can institutions ensure users comprehend model functioning without oversimplifying critical details? Moreover, while AI models might perform well on historical data, they may struggle to generalize in real-world scenarios, leading to overfitting. What processes can ensure that the training data used is diverse and representative of various market conditions?
2. Model Validation: Validating AI models poses unique challenges. The lack of established benchmarks complicates the validation process. The rapidly changing nature of financial markets also means that models may quickly become outdated. Would developing simpler ensemble models offer a more reliable validation benchmark? How often should models be revalidated to stay relevant in changing conditions?
3. Regulatory Risk: Regulatory compliance is a significant concern; for e.g., Basel emphasizes in the Fundamental Review of the Trading Book (FRTB), two approaches for calculating capital requirements: the Standardized Approach (SA) and the Internal Models Approach (IMA). Balancing these approaches while ensuring robust Risk Management presents a significant challenge, moreover introducing AI makes it more challenging. What strategies can firms adopt to ensure that AI-driven assessments remain compliant with regulations? As AI systems become increasingly complex, they may diverge from the standardized methodologies. How can firms reconcile the need for innovative AI solutions with compliance to standardized risk measures? Furthermore, many experts believe that it may take regulators 10-20 years to fully adapt to the rapid advancements in AI technology and its implications for Risk Management.
4. Reproducibility Risk: In AI models, the challenges associated with generating the same outputs when using identical inputs across different executions of the model can arise from various factors, such as changes in model parameters, variations in the underlying data, or even differences in computational environments. As Risk Managers rely more on AI-driven decision-making, the inability to reproduce results can undermine confidence in the model’s reliability and effectiveness. How can organizations establish protocols and frameworks to ensure that AI models produce consistent and reliable results, thereby enhancing trust in their Risk Management practices?
5. Loss of Contextual Understanding: AI often focuses on data-driven patterns and may overlook qualitative factors or contextual knowledge. For instance, how can models account for sudden geopolitical crises that impact market stability, potentially leading to blind spots in risk assessments?
6. Integration of Multi-Dimensional Risks: AI models may struggle to integrate various interrelated risks, such as credit, market, and operational risks. Given that risk management often requires a holistic view, how can AI’s compartmentalized approach be adjusted to better understand complex interactions?
7. Ethical and Moral Dilemmas: AI decision-making raises ethical concerns, especially in sensitive areas like credit scoring or insurance underwriting. How can firms navigate the risk of producing technically valid but ethically questionable outcomes that may disadvantage specific groups?
8. Integration with Legacy Systems: Many firms rely on outdated systems that struggle to integrate with advanced AI technologies. What investments in infrastructure are necessary to overcome these integration challenges?
9. Human-AI Collaboration Challenges: Effective Risk Management often requires a collaborative approach between humans and AI systems. However, if the relationship is not well-defined, it can lead to confusion regarding roles and responsibilities. If roles and responsibilities are unclear, how can organizations prevent decision-making bottlenecks or errors that undervalue human intuition?
Envisioning a practical Future of AI-driven Risk Management
The future of AI in Risk Management holds great promise, especially as we explore ways to overcome the drawbacks and integrate AI intelligently rather than adopting an all-or-nothing approach. By blending AI-driven tools with human oversight, we can address challenges and capitalize on the advantages of both. A practical example is the use of AI-powered chatbots to enhance risk analysis and reporting while mitigating many common concerns associated with AI.
(Throughout this section, specific AI drawbacks that can be overcome are indicated in brackets.)
Imagine a scenario where a bespoke risk report is needed. Instead of relying on lengthy traditional methods, users could engage effortlessly with a chatbot integrated into existing Risk Management systems. This intelligent assistant would interact with users, gathering essential inputs—like market assumptions and specific risk factors—(Model Risk) and then communicate directly with legacy risk systems to generate customized reports almost instantly. By standardizing interactions and outputs (Model Validation and Regulatory Risk), the chatbot can ensure that results are consistent each time similar inputs are provided (Reproducibility Risk). This capability enhances trust in the model’s reliability and effectiveness.
Utilizing legacy risk systems (Integration with Legacy Systems), the chatbot could fetch the necessary data and pass it through established frameworks to generate a summarized output (Integration of Multi-Dimensional Risks). This output would distill complex data into key indicators (Human-AI Collaboration Challenges and Loss of Contextual Understanding), providing a more accessible format than traditional reports. For example, the chatbot could present insights on key performance metrics related to various risks, such as the Probability of Default (PD) in Credit Risk, Key Risk Indicators (KRIs) in Operational Risk, the Liquidity Coverage Ratio (LCR) in Liquidity Risk, or Value-at-Risk (VaR) in Market Risk.
With real-time calculations and tailored insights, the chatbot would empower users to make informed decisions swiftly, enhancing their understanding of their financial landscape. Envisioning an AI-driven future, the integration of intelligent chatbots in risk management—combined with transparent, reproducible, and standardized models built with regulatory compliance (Model Risk, Model Validation, Reproducibility, and Regulatory Risk)—could transform how organizations interact with risk analysis, enabling faster and more effective decision-making.
Conclusion
AI is undeniably reshaping industries across the globe, with Financial Risk Management poised to follow suit. The technology offers transformative possibilities, enhancing decision-making and optimizing risk assessments by empowering firms to harness vast data for real-time insights and proactive strategies. Yet, challenges—such as model risk, validation complexities, and ethical considerations—underscore the need for a thoughtful, well-informed approach to AI adoption.
Looking towards the future, one must ask: will organizations fully embrace the AI revolution in Risk Management, or will they lean toward a model that upholds human oversight and ethical stewardship?
For now, a hybrid approach that combines intelligent automation with human expertise and ethical safeguards appears the most prudent path forward. The coming years will be pivotal in shaping the role of AI as a strategic partner in Risk Management, determining how technology and human insight can best collaborate to create a robust, responsible framework for the future.